LLM as a Judge vs. Human Evaluation
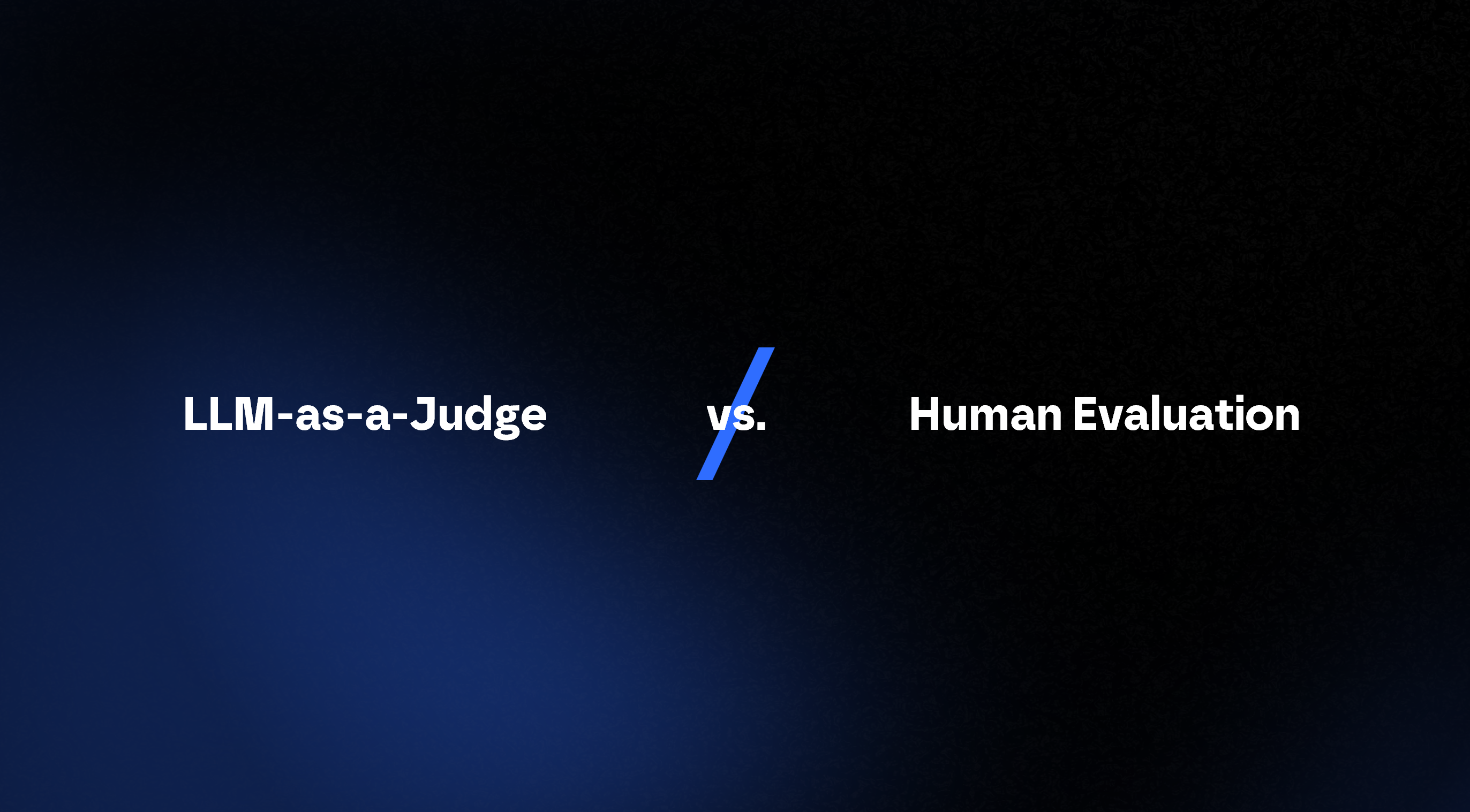
In the rapidly evolving landscape of AI, we're witnessing a paradigm shift in how we evaluate and validate LLM-generated content. The traditional reliance on human experts for quality assurance is being challenged by a compelling alternative: Large Language Models (LLMs) as judges. At Root Signals, we find this development both fascinating and potentially disruptive to the way GenAI is assessed.
Let's start with what's familiar. Human evaluation, particularly by domain experts, has long been the gold standard for assessing AI outputs. Whether it's lawyers reviewing AI-generated contracts or doctors scrutinizing medical summaries, we've traditionally leaned heavily on human expertise. Why? Because humans bring:
However, human evaluation is not without its drawbacks. Humans are prone to inconsistencies, bias, and fatigue, which can lead to errors over time. It’s also slow and costly, making it difficult to scale as LLM systems grow more complex. The bottleneck becomes clear: as GenAI expands, so too does the need for more efficient evaluation methods.
The idea of using LLMs to evaluate other AI-generated outputs is gaining significant traction, and for good reason. LLMs offer several key advantages:
The research paper "LLMs instead of Human Judges? A Large Scale Empirical Study across 20 NLP Evaluation Tasks" reveals that Large language models, when fine-tuned for specific tasks such as automated reasoning and code generation, can perform at or above human levels in certain specialized areas. This shift toward AI-driven evaluation is not just about speed, but also about enhancing the accuracy and reliability of assessments.
Platforms like Root Signals are pioneering the use of LLM-based evaluation methods. But they're not just replacing human evaluators with machines—they're transforming the evaluation process entirely.
By harnessing the power of LLMs, GenAI teams are now able to:
This approach allows for evaluations that are more scalable, efficient, and consistent, offering a glimpse into what the future of AI assessment could look like.
This brings us to the contentious question: Are we nearing a point where LLMs could outperform human experts in evaluation tasks? Data increasingly suggests that, in certain domains, the answer may be yes. For example, an LLM trained on thousands of legal documents and precedents could potentially spot inconsistencies or errors in contracts more efficiently than a human lawyer.
LLMs are particularly effective at handling repetitive, large-scale document review tasks in the legal sector, offering speed and accuracy that even seasoned professionals may struggle to match. This doesn’t mean that human expertise will be rendered obsolete, but it does suggest that AI can augment human abilities in ways previously unimaginable.
As we believe, the most pragmatic solution lies in a hybrid approach that combines the strengths of both human experts and LLMs. This hybrid model could look like:
This collaboration between AI and human expertise will allow organizations to achieve a level of efficiency and accuracy that neither can reach alone. A hybrid model offers the best of both worlds—speed and scale from LLMs, and strategic insight from human experts.
The rise of LLM-as-Judge technologies, as exemplified by platforms like Root Signals, marks more than just an incremental improvement in LLM evaluation. It's a fundamental shift in how we assess LLM outputs at scale. While human expertise remains invaluable, the efficiency, consistency, and scalability of LLM-based evaluation cannot be ignored.
The future of evaluation will likely involve a symbiosis of human insight and LLM efficiency. The question isn't whether LLMs will replace human evaluation, but rather how we can best leverage both to unlock new possibilities for GenAI capabilities and reliability. At Root Signals, we see this as an exciting opportunity to rethink the future of AI assessment—and we are ready to be part of that transformation.